Moments and their Interpretation
Mathematical Treatment
This is part of the moments math collection.Moments
Moments are defined for every distribution. These are generalized functions which find use in a variety of statistical experimental data analysis. In particular, we briefly elucidate how various moments are used to characterize the shape of a distribution.
Finally, we note in passing that a distribution may be totally described via its moments.
- Moments
The expectation is called the moment of the random variable about the number
Initial Moments
Moments about zero are often referred to as the moments of a random variable or the initial moments.
The moment satisfies the relation:
Central Moments
When , then the moment of the random variable about is called the central moment.
The central moment satisfies the relation:
Remark: We note that and for random variables.
Central and Initial Moment Relations
We have:
Also we note that, for distributions symmetric about the expectation, the existing central moments of even order are zero.
Condition for Unique Determinacy
A probability distribution may be uniquely determined by the moments provided that they all exist and the following condition is satisfied:
Additional Moments
Absolute Moments
The absolute moment of about is defined by:
The existence of a moment or implies the existence of the moments and of all orders
Mixed Moments
We note in passing that the mixed second moment is better known as the covariance of two random variables and is defined as the central moment of order (1+1):
Moment Interpretations
We note the following:
- The first initial moment is the expectation.
- The second central moment is the variance.
- The third central moment is related to the skewness.
- The fourth central moment is related to the kurtosis.
- Skewness
A measure of lopsidedness, for symmetric distributions. (a.k.a asymmetry coefficient)
Mathematically:
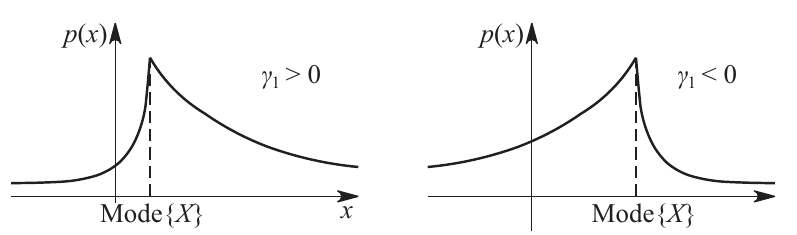
- Kurtosis
A measure of the heaviness of the tail of the distribution, compared to the normal distribution of the same variance. (a.k.a excess, excess coefficient) Essentially a measure of the tails of the distribution compared with the tails of a Gaussian random variable. (Florescu and Tudor 2013)
Mathematically (Polyanin and Chernoutsan 2010):
References
Florescu, I., and C.A. Tudor. 2013. Handbook of Probability. Wiley Handbooks in Applied Statistics. Wiley. https://books.google.co.in/books?id=2V3SAQAAQBAJ.
Polyanin, A.D., and A.I. Chernoutsan. 2010. A Concise Handbook of Mathematics, Physics, and Engineering Sciences. CRC Press. https://books.google.co.in/books?id=ejzScufwDRUC.